Tech
Is Forecasting A Part Of Data Science?
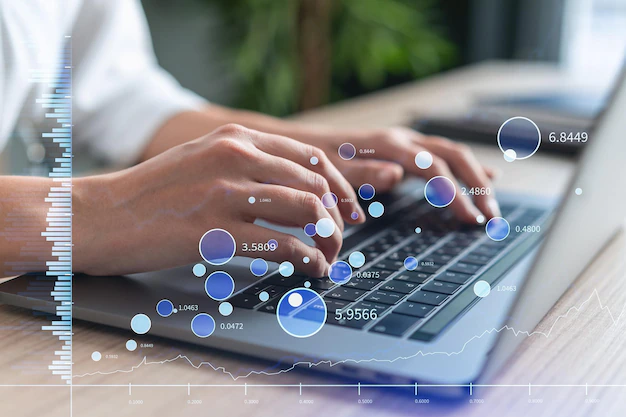
Forecasting is the act of predicting or estimating (a future event or trend). Forecasting determines what will happen for businesses and analysts by evaluating what has happened in the past and what is happening today.
In data science, forecasting is the technique of forecasting or estimating future occurrences based on past and present data, most typically through trend analysis.
Data science is a broad phrase that involves anything from processing traditional or large amounts of data to explaining trends and predicting behavior.
You can use traditional approaches such as regression and cluster analysis and unconventional machine learning techniques in data science.
Data Science is a broad area, and we hope you get a better sense of how all-encompassing and entwined it is with human existence after reading this article. Forecasting is an essential part of data science, and we will elaborate on how.
Forecasting, or making predictions, is an important part of every company’s decision-making process if it wants to stay in business.
This is because today’s decisions, based on projections, influence tomorrow’s success.
What are the traditional forecasting methods in Data Science?
Traditional forecasting methods include traditional statistical forecasting approaches such as linear regression analysis, clustering, logistic regression analysis, time series, and factor analysis.
The results of each of them are fed into the more advanced machine learning analytics, but let’s look at them separately first.
Some of these approaches are also referred to as machine learning in the data science business; however, this article focuses on newer, smarter, and superior methods, such as deep learning. To know more about forecasting in data science, you can sign up for online data science courses from Great Learning.
Linear Regression:
The linear regression model is used in data science to quantify causal links among the variables included in the investigation.
As an example, consider the link between housing prices, house size, neighborhood, and year built.
If you have the necessary information, the model will produce coefficients that will allow you to anticipate the price of a new house.
Logistic Regression
Because you cannot express all interactions between variables as linear, data science uses methods such as logistic regression to develop non-linear models.
Logistic regression is based on 0s and 1s. Companies, for example, use logistic regression algorithms to filter job prospects during the screening process.
Cluster Analysis
When the observations present in the data form groups based on certain criteria, this exploratory data science approach is used.
Cluster analysis considers that certain observations have commonalities and promotes the discovery of additional relevant predictors not included in the initial data conceptualization.
You may make a similar contrast between predictive analytics and their methodologies: classical data science approaches vs. Machine Learning. One is concerned with traditional data, while the other is concerned with big data.
What is the difference between forecasting and predictive analysis?
Predictive analytics appears to be a game-changer for many businesses and demand planners.
Others see it as a more advanced variant of standard demand planning. Explanatory data analysis is the foundation of predictive analytics in data science.
Unlike traditional forecasting, which is based on statistics and uses level, trend, and seasonality data to predict results, predictive analytics is based on consumer behavior. It may employ explanatory factors to predict outcomes.
The basic difference between forecasting and predictive analysis is that forecasting is requirements-based, with limited demand factors, and forecasts sales. It also tells you what to order and is used when the relationship between variables is strong, and lastly, it provides output to one specific question.
On the other hand, predictive analysis is opportunity-oriented, with many factors; it predicts drivers and tells us why the consumer buys.
You can also use it to assess relationships between unknown variables, and lastly, it provides multiple insights and feasible solutions for the entire business.
Uses of traditional forecasting methods
The data scientist uses traditional forecasting methods, but it is to be kept in mind that this title also refers to someone who uses machine learning techniques for analytics. Much of the work is transferred from one approach to the next.
On the other hand, a data analyst is a person who conducts sophisticated sorts of studies that explain existing patterns in data and ignores the fundamental element of predictive analytics.
What are the programming languages in data science?
Knowing a programming language allows the data scientist to create programs that can do certain tasks.
The most significant advantage of programming languages is reusing programs designed to do the same activity several times.
When paired with SQL, R, Python, and MATLAB cover most of the tools used when working with traditional data, business intelligence, and traditional data science.
R and Python are the two most popular data science tools across all sub-disciplines. Their main benefit is that they can alter data and are compatible with various data and data science software systems.
They are appropriate for mathematical and statistical computations, but they are also flexible.
Of course, R and Python are used to manage big data in data science, but professionals in this field are typically fluent in other languages such as Java or Scala. These two are quite helpful when merging data from many sources.
Conclusion
One of the difficulties in forecasting is determining the number of recent occurrences you should consider when making predictions.
This also relies on whether you are deciding on the near or distant future. However, for multiple reasons, it is still a favorite method for many data analysts, and they prefer the traditional model of forecasting over newer methods such as predictive analysis.
Predictive analysis is concerned with predicting the outcomes of unobserved data. Forecasting is a sub-discipline of prediction in which we make future predictions based on time-series data.
For more information regarding data science & analytics, I recommend a PGP in data science & business analytics from Great Learning. They provide excellent online courses for the learner’s convenience.
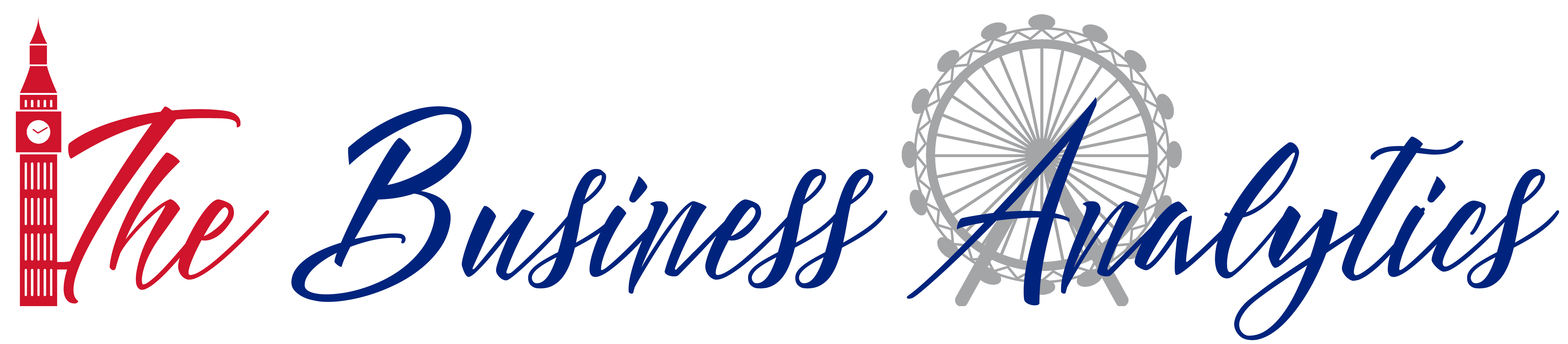
Artificial Intelligence
YouTube Introduces ‘Hype,’ A New Tool to Boost Smaller Creators’ Reach
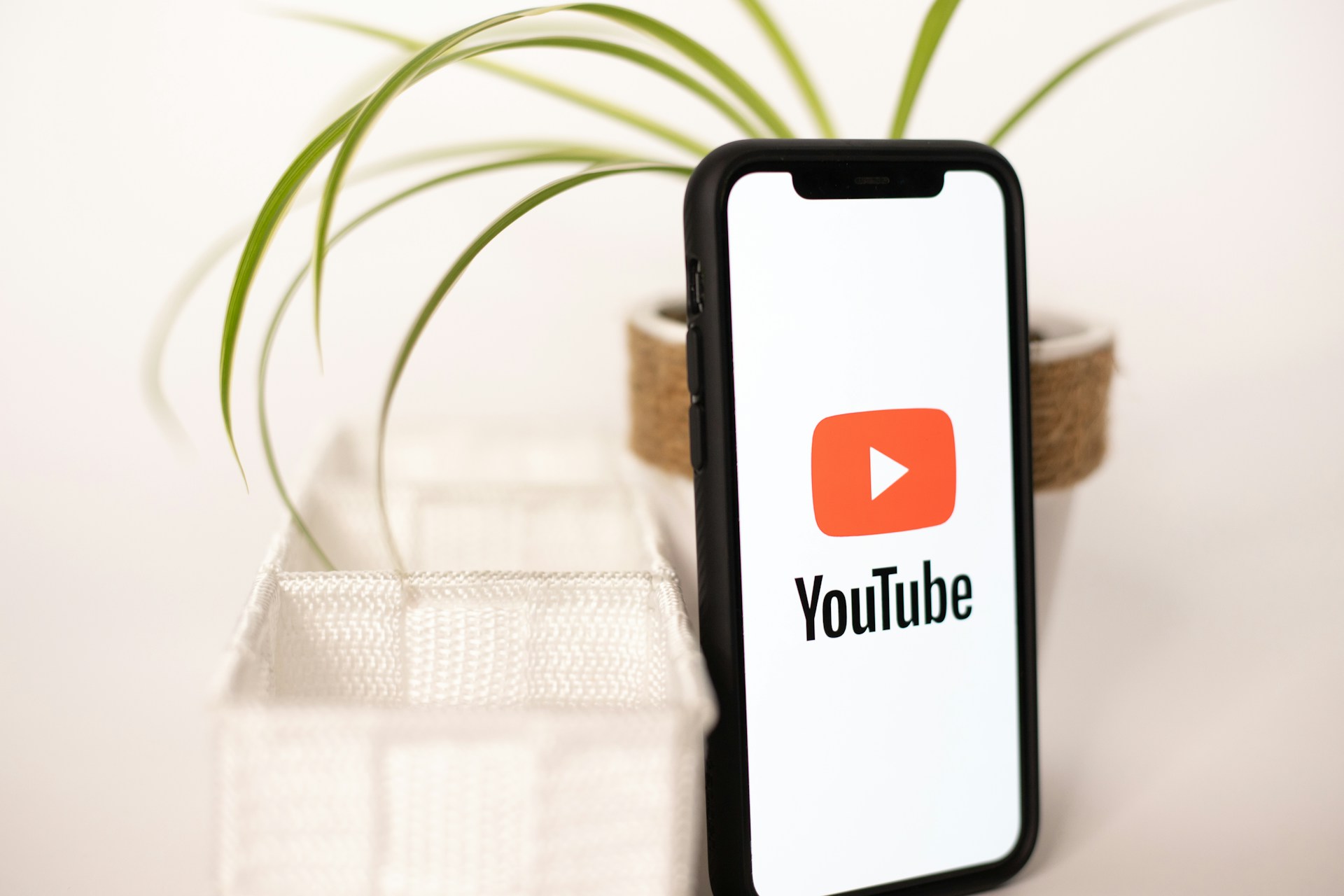
YouTube has rolled out a new feature called Hype, aimed at helping smaller creators grow their audience without solely relying on the platform’s recommendation algorithm, search, or collaborations. Announced at the Made on YouTube event, this feature allows a creator’s fans to directly contribute to the visibility of their videos.
How does the Hype Feature work?
fans can click the Hype button located just below the like button on a video. This feature is available only for creators with fewer than 500,000 subscribers. As more viewers engage with the Hype button, the video moves up on the top 100 hyped videos leaderboard, giving it a chance to reach a wider audience. To prevent users from overusing the feature to unfairly promote their favorite creators, fans are currently limited to three hypes per week.
YouTube developed Hype after recognizing that passionate fans wanted a more active role in their favorite creators’ growth. In the future, the platform plans to allow users to purchase additional hypes, introducing a new revenue stream. However, YouTube hasn’t disclosed what percentage it will take from these purchases. Currently, on purchases like Super Thanks, YouTube takes 30% of the revenue, sharing the remaining 70% with creators.
As creators receive hype, they’ll earn points that help them climb their country’s weekly leaderboard. To level the playing field for smaller creators, YouTube will also offer a bonus multiplier to channels with fewer subscribers, helping them compete with larger channels. Top hyped videos will also be awarded a special badge to highlight their achievement.
According to Bangaly Kaba, YouTube’s Director of Product Management, Hype gives fans a new way to show their support for emerging creators and help boost their latest videos. In the future, creators will be able to see exactly who hyped their content.
During the first four weeks of beta testing in Turkey, Taiwan, and Brazil, the Hype feature was used over 5 million times across more than 50,000 channels. The largest group of users during the beta, making up over 30%, were aged 18 to 24.
Health
Digital Transformation: the importance for Pharma and MedTech Companies
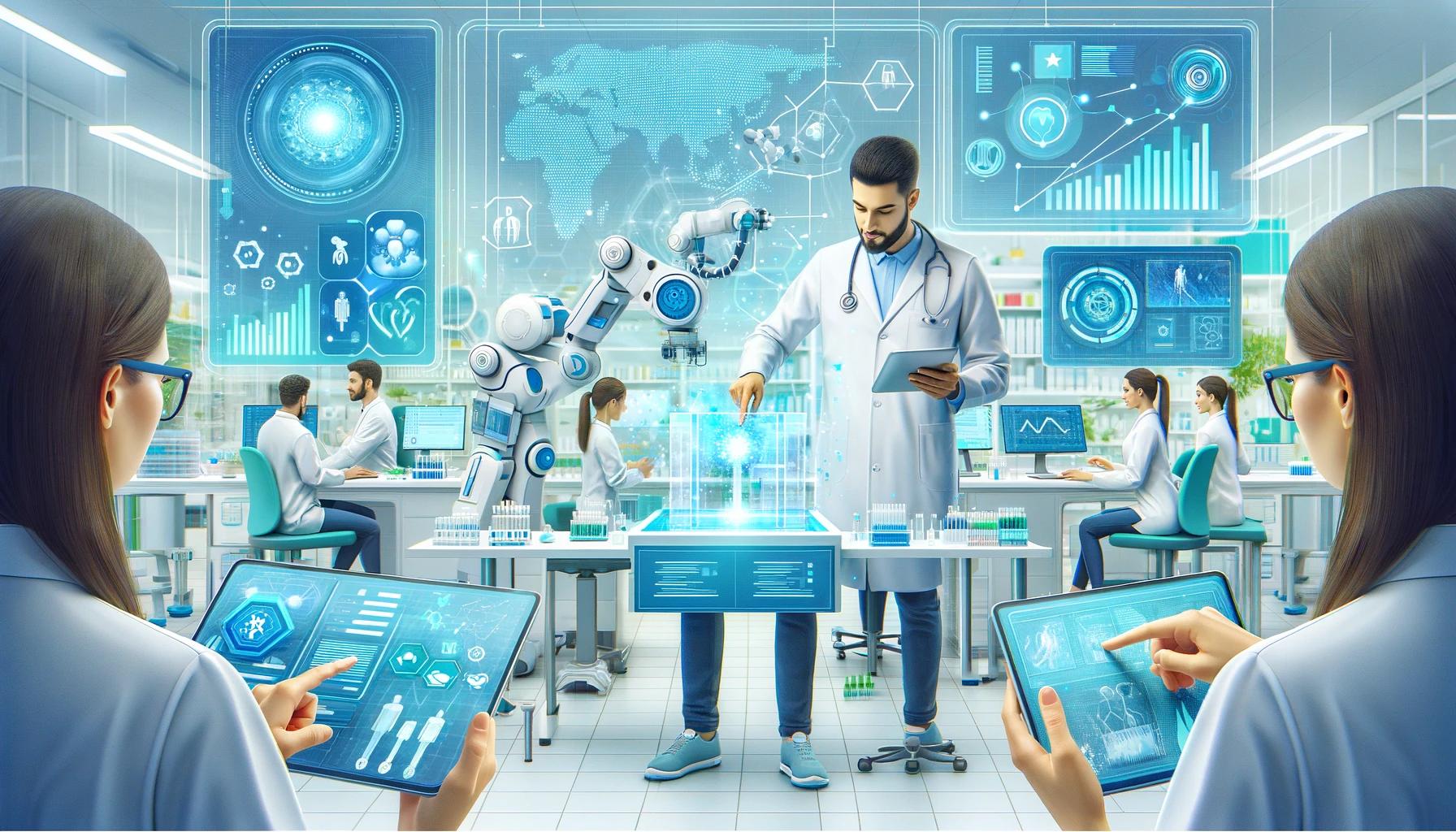
Digital transformation refers to integrating digital technology into all areas of a business, fundamentally changing how companies operate and deliver value to customers. This transformation is crucial for staying competitive and innovative in the pharmaceutical and MedTech industries.
Pharma companies are increasingly recognizing the importance of digital innovation (among which AI is playing an increasingly important role) to enhance manufacturing processes, streamline operations, and improve patient outcomes. The COVID-19 pandemic has accelerated the adoption of digital tools, highlighting their potential to expedite drug development and bring life-saving treatments to market more efficiently.
As healthcare continues to evolve, embracing technology and investing in digital transformation in pharma will be essential for companies aiming to stay ahead in this dynamic sector.
Setting the vision
The first step in digital transformation for pharma companies is to set a clear vision. This involves answering essential questions such as:
- What are the company’s long-term goals?
- How can digital tools help achieve these goals?
- What specific areas need improvement?
By addressing these questions, companies can better articulate their needs to service providers and move forward with a well-defined plan. Setting this vision is crucial for aligning the entire organization towards common objectives and ensuring that every digital initiative contributes to the overall strategy. For instance, if a company aims to enhance its manufacturing efficiency, it should explore digital innovation solutions like AI-driven automation and predictive analytics.
Furthermore, understanding the trend of pharma landscape in 2024 can provide valuable insights into emerging technologies and practices that could be integrated into the company’s digital strategy. For example, digital transformation in pharma manufacturing often involves incorporating advanced technology to streamline processes, reduce costs, and improve product quality.
Key components of digital transformation
Semi-digital vs. fully digital transformation
Many pharma companies are currently stuck in a semi-digital phase. This stage involves processes where documents are manually scanned and then saved as digital versions.
While this represents a step towards digital innovation, it falls short of a true digital transformation. Real digital transformation in pharma and MedTech companies requires eliminating manual intervention and automating the entire process flow. For instance, advanced systems can automate document creation, approval, and updates, ensuring that all relevant files are seamlessly integrated and maintained.
By moving beyond semi-digital practices, companies can fully harness the benefits of digital technology, improving efficiency and compliance. This shift is crucial for staying competitive in an industry increasingly driven by digital transformation in pharma marketing and manufacturing.
Computer system validation (CSV)
In the context of digital transformation in pharma, Computer System Validation (CSV) is a crucial element for ensuring that software systems perform as intended and comply with regulatory standards. The validation process, however, can be extensive and complex, often requiring significant time and resources.
To streamline this, many pharma companies are turning to external CSV consultants or leveraging solutions that adhere to the GAMP5 validation approach. This method not only simplifies the validation process but also aligns with industry best practices, ensuring compliance and operational efficiency.
By adopting such strategies, companies can better navigate the challenges of digital transformation, particularly in the pharma manufacturing and pharma marketing sectors, setting the stage for enhanced productivity and regulatory compliance.
Role of AI in digital transformation
Artificial Intelligence (AI) is transforming the pharmaceutical and MedTech industries by enhancing efficiency, accuracy, and innovation. AI streamlines drug discovery optimizes manufacturing processes, and improves patient outcomes, giving companies a competitive edge.
In drug development, AI accelerates the discovery process by analyzing large datasets to identify potential drug candidates faster and more accurately. It optimizes clinical trials by selecting suitable patients and predicting trial outcomes, leading to faster and more reliable results. In manufacturing, AI-driven automation and predictive analytics improve production efficiency, reduce downtime, and ensure product quality by identifying real-time anomalies.
AI is also revolutionizing supply chain management by improving decision-making, reducing inventory levels, and minimizing out-of-stock scenarios. Integrating solutions like AI for smart pharmaceutical inventory management allows companies to achieve substantial savings. Moreover, AI enhances personalized patient care by analyzing data to provide tailored treatment recommendations and predict medication responses, thus improving healthcare quality.
Implementation and compliance considerations
Accessibility and affordability
Digital transformation in pharma is not just about adopting the latest technologies; it also involves ensuring that these technologies are accessible and affordable for all stakeholders involved. To achieve this, pharma companies must prioritize solutions that are easy to implement across various locations and cost-effective.
Cloud-based platforms are particularly suitable as they offer global implementation capabilities without the need for site-specific installations. This approach not only streamlines operations but also significantly reduces overhead costs, making it a viable option for both large enterprises and startups. By leveraging cloud technology, companies can ensure that their digital transformation efforts are both inclusive and financially sustainable, aligning with the broader goals of digital innovation and enhanced healthcare delivery.
Implementation and transformation time
The time required for a complete overhaul often raises concerns among pharma companies. However, with the right partner, this process can be both swift and seamless.
The key lies in selecting a vendor that provides end-to-end solutions and offers additional support for data import, integration, and training. This comprehensive approach not only accelerates the transition but also ensures that internal IT and QA teams are not overwhelmed by the demands of the transformation.
By leveraging such partnerships, companies can effectively navigate the complexities of digital transformation, thereby enhancing their operational efficiency and competitiveness in the healthcare sector.
Regulatory compliance
In the realm of digital transformation in pharma, regulatory compliance remains a critical consideration. Both pharma companies and MedTech industries must adhere to stringent regulatory standards to ensure the safety and efficacy of their products. Therefore, sourcing a digital solution that meets all compliance criteria is essential.
Opting for 21 CFR Part 11-compliant software can help companies overcome regulatory hurdles by ensuring that electronic records and signatures are trustworthy, reliable, and equivalent to paper records. This is particularly important in the context of digital innovation and healthcare, where maintaining data integrity and security is paramount.
Additionally, the implementation of compliant software solutions can streamline manufacturing processes, reduce the risk of non-compliance, and facilitate smoother audits. As technology continues to evolve, staying ahead of compliance requirements will be crucial for maintaining operational efficiency and ensuring patient safety.
Conclusion
Championing an end-to-end digital transformation project requires careful planning, vendor selection, and execution. Partnering with an experienced service provider can significantly ease the transition from being digital to being digital.
This approach not only streamlines the implementation process but also ensures that the transformation aligns with industry standards and regulatory requirements. By leveraging the expertise of a seasoned provider, pharma companies can focus on core activities while seamlessly integrating digital innovation into their operations.
This strategic partnership is crucial for navigating the complexities of digital transformation in pharma manufacturing and healthcare, ultimately enhancing operational efficiency and accelerating time-to-market for new treatments.
Artificial Intelligence
Galaxy AI to Expand to Mid-Range Galaxy A Phones in 2024
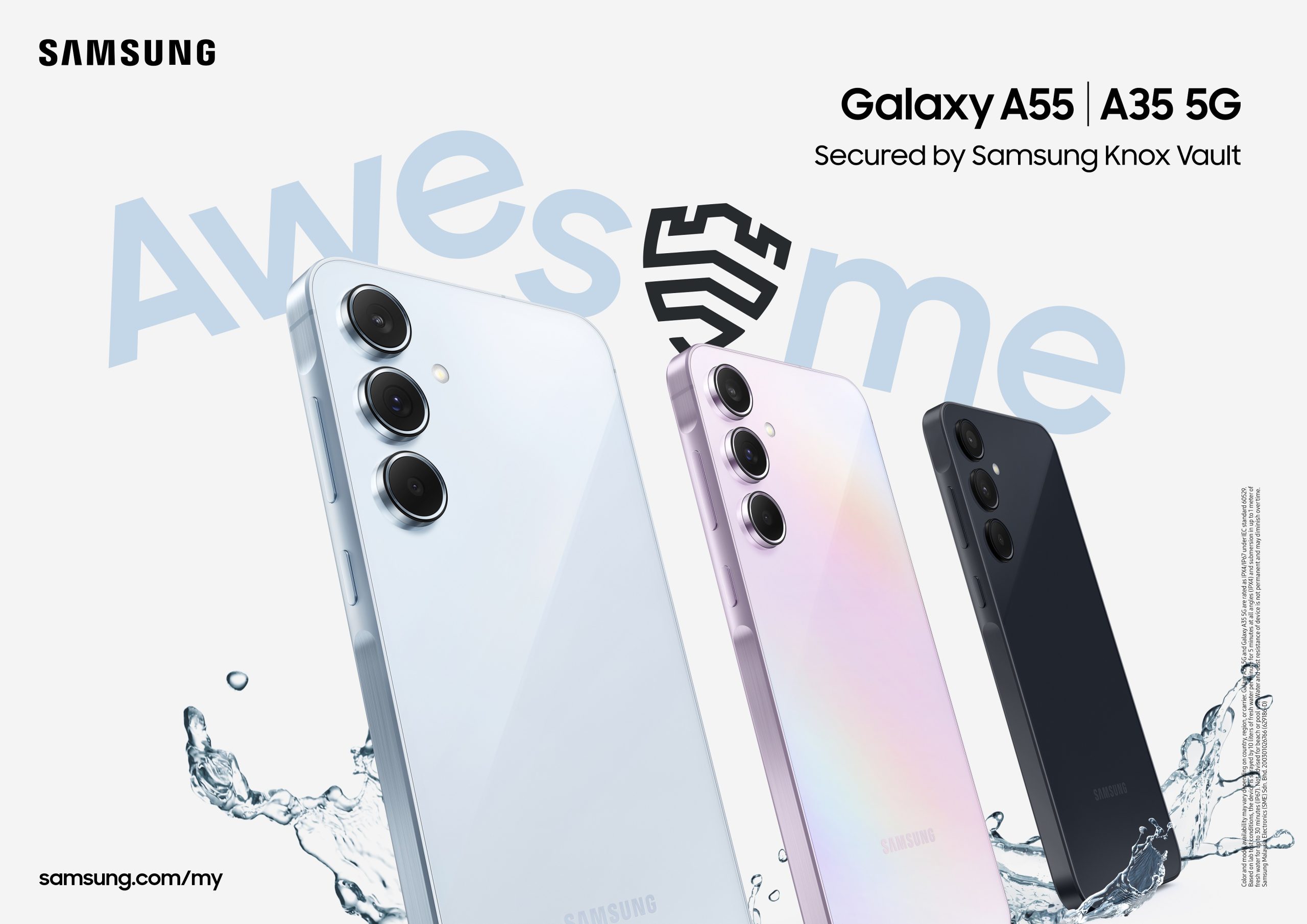
Exciting news for Galaxy A phone users! Samsung is set to bring its innovative Galaxy AI technology to select mid-range Galaxy A models in 2024. This expansion will start with the Galaxy A35 and Galaxy A55, according to sources from SamMobile.
Limited Galaxy AI Features for Galaxy A35 and A55
While this is a significant move, it’s important to note that not all Galaxy AI features will be available on these mid-range devices. Samsung has yet to specify which AI tools will be included, but features requiring substantial on-device processing power will likely be omitted.
The rollout of Galaxy AI to the Galaxy A35 and A55 is expected to happen through the One UI 6.1.1 update, which could arrive as early as this month or next. However, there is no confirmed release date.
Current Availability and Limitations
As of now, the most affordable Galaxy AI-enabled phone available is the Galaxy S23 FE. Even this model does not include all the AI features found in the original Galaxy AI suite for the Galaxy S24 series, such as Instant Slow-Mo.
Despite hardware similarities, older Galaxy A models like the Galaxy A54, which shares the Exynos 1380 SoC with the Galaxy A35, are not confirmed to receive Galaxy AI. Samsung appears to be focusing on models released in 2024 and later.
Samsung’s AI Ambitions
At Unpacked 2024, Samsung announced its ambition to extend Galaxy AI tools to over 200 million Galaxy devices, including phones, tablets, and wearables. This expansion to mid-range Galaxy A phones is a part of that strategy, aiming to democratize advanced AI features across a broader range of devices.
Summary
Samsung’s Galaxy AI is set to arrive on mid-range Galaxy A phones, starting with the Galaxy A35 and A55, through the One UI 6.1.1 update. While not all AI features will be available, this marks a significant step in making advanced AI technology more accessible. Stay tuned for further updates on the rollout and specific features.
-
Business2 years ago
The Most Efficient Ways To Use The Best 6 Business Keynote Presentations
-
Apps and Software2 years ago
Starbucks Partner Hours App Login Guide
-
Entertainment1 year ago
15 Best IPTV Service Providers in the UK 2023
-
Economy3 years ago
What does it mean to Dream About Pennies?
-
Entertainment3 years ago
10 Best Free Video Player Apps For Apple TV
-
Entertainment1 year ago
How To Enhance Your Viewing with Video Subtitle Support
-
Apps and Software2 years ago
How to Download Full Crack Version of Microsoft Office 2013 Torrent
-
Business1 year ago
How to Automate Desk Booking Systems at a Coworking Space